Topic: Post Disaster Damage Assessment Using Ultra-High-Resolution Aerial Imagery with Semi- Supervised Transformers

Abstract
In post-disaster scenarios, efficient preliminary damage assessments (PDAs) are crucial for a resilient recovery. Traditional door-to-door inspections are time-consuming, potentially delaying resource allocation. To automate PDAs, numerous studies propose frameworks utilizing data from satellites, UAVs, or ground vehicles, processed with deep CNNs. However, before such frameworks can be adopted in practice, the accuracy and fidelity of predictions of damage level at the scale of an entire building must be comparable to human assessments. Our proposed framework integrates ultra-high-resolution aerial (UHRA) images with state-of-the-art transformers, showcasing that semi-supervised models, trained with vast unlabeled data, surpass existing PDA frameworks. Our experiments explore the impact of unlabeled data, varied data sources, and model architectures.
Highlights
- Best Model (Semi-ViT) achieved 88% accuracy in 5-class classification, surpassing the accuracy of the current state-of-the-art model.
- Transformer outperformed CNN, achieving 73% accuracy surpassing human level accuracy.
- With Just 268 labelled images, the Semi-ViT model achieved 81% accuracy
- UHRA enhances the model’s ability to generalize to different datasets and improves its performance in distinguishing between classes.
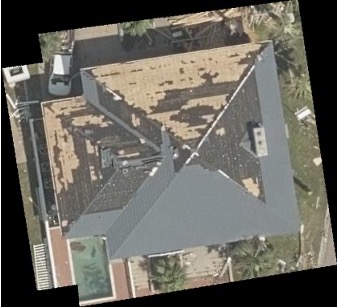
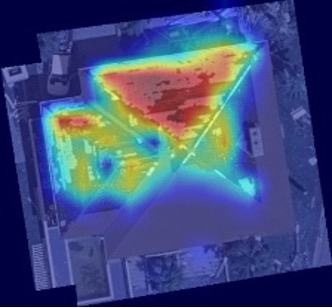
Please Cite:
Singh, D.K.; Hoskere, V. Post Disaster Damage Assessment Using Ultra-High-Resolution Aerial Imagery with Semi-Supervised Transformers. Sensors 2023, 23, 8235. https://doi.org/10.3390/s23198235