Post Disaster Damage Assessment Using Ultra-High-Resolution Aerial Imagery with Semi-Supervised Transformers (2023)
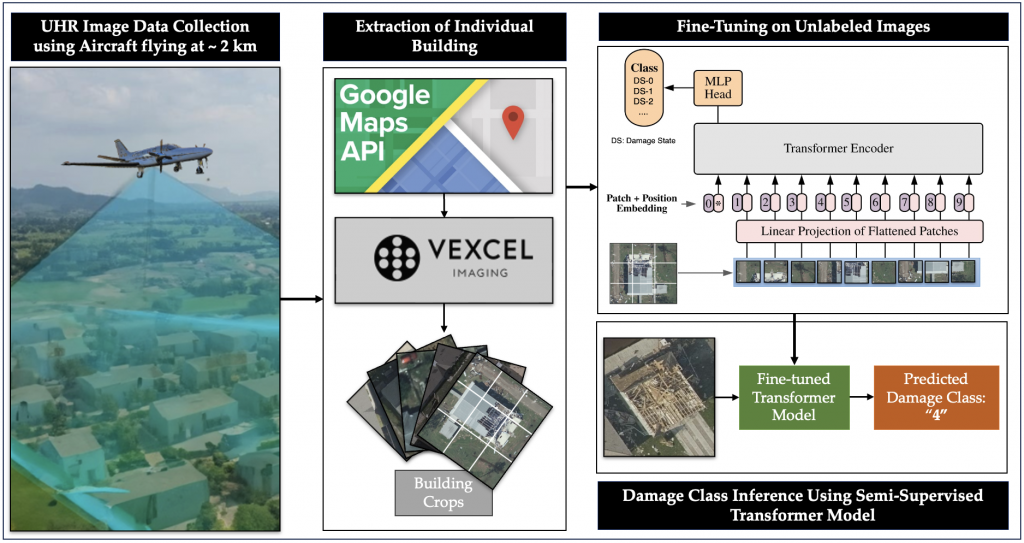
Deepank Kumar singh and Vedhus Hoskere
In post-disaster scenarios, efficient preliminary damage assessments (PDAs) are crucial for a resilient recovery. Traditional door-to-door inspections are time-consuming, potentially delaying resource allocation. To automate PDAs, numerous studies propose frameworks utilizing data from satellites, UAVs, or ground vehicles, processed with deep CNNs. However, before such frameworks can be adopted in practice, the accuracy and fidelity of predictions of damage level at the scale of an entire building must be comparable to human assessments. Our proposed framework integrates ultra-high-resolution aerial (UHRA) images with state-of-the-art transformers, showcasing that semi-supervised models, trained with vast unlabeled data, surpass existing PDA frameworks. Our experiments explore the impact of unlabeled data, varied data sources, and model architectures.
Unpaired Image-to-Image Translation of Structural Damage (2023)
Subin Varghese and Vedhus Hoskere
Paper
EIGAN, a novel cycle-consistent adversarial network (CCAN) architecture for generating realistic synthetic images of damaged infrastructure from undamaged images. The lack of diverse datasets is a critical challenge for training robust and generalizable deep neural networks for damage identification. To address this, EIGAN is proposed as a means of data augmentation to improve network performance. EIGAN outperforms other established CCAN architectures and retains the geometric structure of the infrastructure.
Physics‐based Graphics Models in 3D Synthetic Environments enabling Autonomous Vision‐based Structural Inspections (2021)
Vedhus Hoskere, Yasutaka Narazaki, and Billie F. Spencer Jr.
A high-fidelity nonlinear finite element model is incorporated in the synthetic environment to provide a representation of earthquake-induced damage; this finite element model, combined with photo-realistic rendering of the damage, is termed herein a physics-based graphics models (PBGM). The 3D synthetic environment employing PBGMs is shown to provide an effective testbed for development and validation of autonomous vision-based post-earthquake inspections that can serve as an important building block for advancing autonomous data to decision frameworks.
Post-Earthquake Inspections with Deep Learning-based Condition-Aware Models (2018)
Vedhus Hoskere, Yasutaka Narazaki, Tu Hoang, and Billie F. Spencer Jr.
We propose a new framework to generate deep-learning based condition-aware models that combine information about the type of structure, its various components and the condition of each of the components, all inferred directly from sensed data. Condition-aware models can be used as the basis to obtain higher level assessments on the structure using the statistics and type of the defects observed
Synthetic Environments for Vision-based Structural Condition Assessment of Viaducts (2021)
Yasutaka Narazakia, Vedhus Hoskere, Koji Yoshida, Billie F. Spencer, and Yozo Fujino
A unified system is developed to identify and localize structural components and their damage. Synthetic environments are used to produce datasets that are lacking in general. Visual recognition algorithms trained using the synthetic data can perform the structural condition assessment tasks both for synthetic and real-world images. Trained networks are combined to realize the unified system for structural condition assessment.
MaDnet: Multi-task Semantic Segmentation of Structural Materials and Damage in Images of Civil Infrastructure (2020)
Vedhus Hoskere, Yasutaka Narazaki, Tu Hoang, and Billie F. Spencer Jr.
Training separate networks for the tasks of material and damage identification fails to incorporate this intrinsic interdependence between them. We demonstrate that a network that incorporates such interdependence directly will have a better accuracy in material and damage identification. To this end, a deep neural network, termed the material-and-damage-network (MaDnet), is proposed to simultaneously identify material type (concrete, steel, asphalt), as well as fine (cracks, exposed rebar) and coarse (spalling, corrosion) structural damage.
Deep Bayesian Neural Networks for Damage Detection and Model Updating (2019)
Vedhus Hoskere, Brian Eick, Billie F Spencer, Jr, Matthew D Smith, and Stuart D Foltz
This article presents a novel engineering application of structural health monitoring for full-scale civil infrastructure with a method to automatically quantify the damage quantity of interest, that is, the gaps using measured strain data. We propose a framework for damage estimation of full-scale civil infrastructure in general and miter gates in particular, leveraging recent advances in deep Bayesian learning
Vision-Based Modal Survey of Civil Infrastructure Using Unmanned Aerial Vehicles (2017)
Vedhus Hoskere, Jong-Woong Park, Hyungchul Yoon, and Billie F Spencer, Jr.
In this study, a new approach is presented to facilitate the extraction of frequencies and mode shapes of full-scale civil infrastructure from video obtained by an unmanned aerial vehicle (UAV). This approach addresses directly a number of difficulties associated with modal analysis of full-scale infrastructure using vision-based methods. The proposed approach is evaluated using a story-story shear-building model excited on a shaking table in a laboratory environment, and on a full-scale pedestrian suspension bridge.